1. LightGBM(Light Gradient Boosting Machine)
1.1 LightGBM
LightGBM은 Microsoft에서 개발한 고성능 그래디언트 부스팅 프레임워크입니다.
기존의 그래디언트 부스팅(Gradient Boosting) 방법론을 개선하여 대규모 데이터셋에서의 빠른 학습과 예측을 제공하도록 설계되었습니다.
LightGBM은 특히 대규모 데이터와 복잡한 특징 공간을 가진 문제에서 빠른 처리 속도와 적은 메모리 사용을 강점으로 가집니다.
1.2. LightGBM 발전 역사
GBDT(Gradient Boosting Decision Tree)는 널리 사용되는 기계 학습 알고리즘으로, 여러 효율적인 구현 중 하나가 XGBoost 모델입니다.
이 알고리즘은 특징 차원이 높을 때와 모든 가능한 분할 지점의 정보 이득을 추정해야 할 때 시간이 많이 소요되는 문제를 가지고 있습니다.
이를 극복하기 위해 LightGBM이 개발되었는데, Gradient-based One-Side Sampling (GOSS)과 Exclusive Feature Bundling (EFB)과 같은 알고리즘을 사용했습니다.
이 알고리즘은 특히 전체 데이터셋의 일부분만을 사용하여 더 빠르게 훈련시킬 수 있으며, 고차원의 특성을 효율적으로 처리할 수 있습니다.
1.3 LightGBM 특징
LightGBM은 데이터의 개수가 적을 때(10000개 이하) 과적합이 발생할 수 있습니다. 그럼에도 다양한 장점 때문에 자주 사용됩니다.
※ 장점
1) 고속처리 및 효율성
LightGBM은 병렬 처리 및 데이터 샘플링 최적화를 통해 기존 그래디언트 부스팅 방식보다 훨씬 빠른 학습이 가능하게 합니다.
2) 메모리 효율성을 통한 최적화
연속형 변수에 대해 구간을 만들어줌으로써 계산 과정에서 메모리 사용을 줄입니다.
기존 방식에 비해 높은 처리 속도를 제공하며, 리소스가 제한적인 환경에서도 효율적으로 모델이 운영될 수 있도록 합니다.
3) 결측치 자동 처리
별도의 결측치 처리 과정 없이도, 알고리즘은 결측치가 있는 데이터를 자동으로 인식하고 이를 학습 과정에 사용합니다.
4) 범주형 변수 자동 처리
LightGBM은 범주형 변수를 효과적으로 처리할 수 있습니다.
자료형을 category로 바꾸어 주면 되며, 이 외의 별도의 인코딩 과정이 필요하지 않습니다.
5) 스케일링 불필요
LightGBM은 트리 기반의 모델로서 입력 변수의 스케일에 민감하지 않습니다.
따라서 별도의 스케일링(scaling) 작업 없이도 학습할 수 있습니다.
6) 높은 정확도
LightGBM은 XGBoost와 비교하여 동등하거나 때때로 더 뛰어난 정확도를 제공합니다.
1.4 XGBoost 모델과의 차이
XGBoost (eXtreme Gradient Boosting)는 고성능 그래디언트 부스팅 라이브러리로, 정형 데이터 분석 대회에서 널리 사용되어 왔습니다.
XGBoost는 그래디언트 부스팅의 전통적인 방식을 발전시켜 특히 병렬 처리와 과적합 방지 기능에서 강력한 성능을 발휘합니다.
XGBoost와 LightGBM은 모두 그래디언트 부스팅에 기반한 라이브러리이지만, 주요 차이점이 몇 가지 있습니다.
이들은 대규모 데이터셋에서 뛰어난 성능을 제공하며, 병렬 처리 기능을 통해 훈련 속도를 향상시킵니다.
※ 차이점
1) 성장 방식
XGBoost는 Level-wise 방식을 사용하여 균형 잡힌 트리를 만들어 과적합을 방지하는 반면,
LightGBM은 Leaf-wise 방식을 사용하여 비대칭적인 트리를 빠르게 성장시킬 수 있습니다.
위의 이미지는 Level-wise 성장 방식을 나타낸 것입니다.
Level-wise 성장 방식에서는 모든 노드가 같은 레벨에 있을 때까지 자식 노드를 확장합니다.
Level-wise 성장 방식의 경우 트리가 균형 성장을 하면서 트리의 높이가 최소화되기 때문에 과적합을 방지할 수 있다는 장점이 있지만, 많은 메모리를 사용한다는 단점이 있습니다.
반면, Leaf-wise 접근 방식은 트리의 성장을 최적화하여 가장 큰 손실 감소를 제공하는 노드를 우선적으로 확장합니다.
이 방법은 종종 더 깊은 트리를 만들어 과적합의 우려가 있지만, 적절하게 모델을 구성할 경우 과적합의 위험을 관리하면서도 정확한 모델을 빠르게 구축할 수 있습니다.
2) 속도 및 대규모 데이터 처리
XGBoost는 병렬 처리 기능을 사용하여 동시에 트리를 구축하고 학습 속도를 향상시키는 능력이 있습니다.
그러나 이 과정에서 전체 데이터셋의 모든 특성을 스캔해야 하므로, 매우 큰 데이터셋의 경우 여전히 상당한 계산 비용과 시간이 소요될 수 있습니다.
LightGBM은 히스토그램 기반 분할 방식을 사용하여 이러한 계산 비용을 대폭 줄입니다.
이 방식에서 LightGBM은 데이터의 모든 연속형 변수를 미리 정의된 구간(bin)으로 변환하고, 이 구간 정보를 바탕으로 트리의 분할을 결정합니다.
이 방법은 데이터를 스캔하는 데 필요한 시간을 크게 줄이며, 메모리 사용도 최소화합니다.
3) 범주형 변수 처리
XGBoost는 범주형 데이터를 처리하기 위해 라벨 인코딩, 원 핫 인코딩과 같이 수치형 데이터로 변환을 하여야 합니다.
반면, LightGBM은 범주형 변수의 자료형을 카테고리로 변환하기만 하면 바로 학습이 가능합니다.
2. LightGBM모델 코드
2.1 데이터 로드
import pandas as pd
train = pd.read_csv('신용_train.csv')
print(train.isnull().sum())
train.head(2)
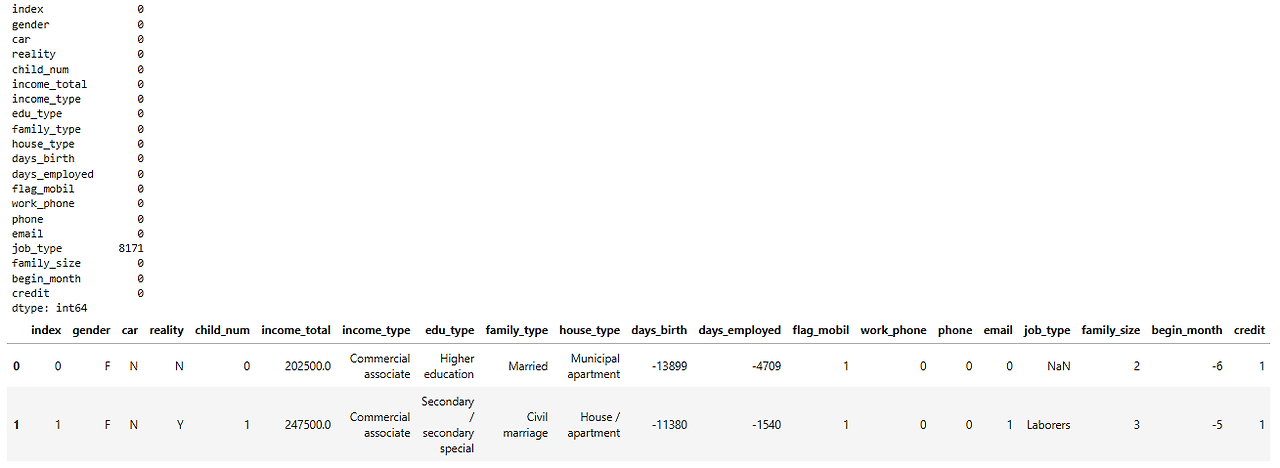
2.2 LightGBM 모델 학습을 위한 데이터 준비 : object인 column을 모두 category로 변경
target = train['credit']
independent = train.drop(['index', 'credit'], axis = 1)
object_cols = [col for col in independent.columns if independent[col].dtype == "object"]
independent[object_cols] = independent[object_cols].astype('category')
from sklearn.model_selection import train_test_split
X_train, X_valid, y_train, y_valid = train_test_split(independent, target, test_size=0.2, random_state=42)
2.3 기본 LightGBM 모델 학습
from lightgbm import LGBMClassifier
# LightGBM 모델 정의 및 학습
base_lgbm = LGBMClassifier(random_state = 42)
base_lgbm.fit(X_train, y_train)
[LightGBM] [Info] Auto-choosing row-wise multi-threading, the overhead of testing was 0.001160 seconds.
You can set `force_row_wise=true` to remove the overhead.
And if memory is not enough, you can set `force_col_wise=true`.
[LightGBM] [Info] Total Bins 781
[LightGBM] [Info] Number of data points in the train set: 21165, number of used features: 17
[LightGBM] [Info] Start training from score -2.107665
[LightGBM] [Info] Start training from score -1.441911
[LightGBM] [Info] Start training from score -0.443162
# 검증점수 확인
print("LGBM 모델 정확도:", base_lgbm.score(X_valid, y_valid))
LGBM 모델 정확도: 0.6993575207860923
2.4 LightGBM 하이퍼파라미터 튜닝
from lightgbm.callback import early_stopping, log_evaluation
# LightGBM 모델 정의
tuning_lgbm = LGBMClassifier(n_estimators=300, max_depth=6, random_state = 42)
# 조기 종료와 학습 로그 출력 콜백 정의
early_stop = early_stopping(stopping_rounds=5)
log_eval = log_evaluation(period=20)
# 모델 학습
tuning_lgbm.fit(
X_train, y_train,
eval_set=[(X_valid, y_valid)],
eval_metric='multi_logloss',
callbacks=[early_stop, log_eval]
)
# 정확도 출력
print("하이퍼파라미터가 튜닝된 LGBM 모델 정확도:", tuning_lgbm.score(X_valid, y_valid))
[LightGBM] [Info] Auto-choosing row-wise multi-threading, the overhead of testing was 0.001140 seconds.
You can set `force_row_wise=true` to remove the overhead.
And if memory is not enough, you can set `force_col_wise=true`.
[LightGBM] [Info] Total Bins 781
[LightGBM] [Info] Number of data points in the train set: 21165, number of used features: 17
[LightGBM] [Info] Start training from score -2.107665
[LightGBM] [Info] Start training from score -1.441911
[LightGBM] [Info] Start training from score -0.443162
Training until validation scores don't improve for 5 rounds
[20] valid_0's multi_logloss: 0.801781
[LightGBM] [Warning] No further splits with positive gain, best gain: -inf
[LightGBM] [Warning] No further splits with positive gain, best gain: -inf
[LightGBM] [Warning] No further splits with positive gain, best gain: -inf
[LightGBM] [Warning] No further splits with positive gain, best gain: -inf
[LightGBM] [Warning] No further splits with positive gain, best gain: -inf
[LightGBM] [Warning] No further splits with positive gain, best gain: -inf
[LightGBM] [Warning] No further splits with positive gain, best gain: -inf
[LightGBM] [Warning] No further splits with positive gain, best gain: -inf
[LightGBM] [Warning] No further splits with positive gain, best gain: -inf
[LightGBM] [Warning] No further splits with positive gain, best gain: -inf
[LightGBM] [Warning] No further splits with positive gain, best gain: -inf
[40] valid_0's multi_logloss: 0.787669
[LightGBM] [Warning] No further splits with positive gain, best gain: -inf
[LightGBM] [Warning] No further splits with positive gain, best gain: -inf
[LightGBM] [Warning] No further splits with positive gain, best gain: -inf
[LightGBM] [Warning] No further splits with positive gain, best gain: -inf
[LightGBM] [Warning] No further splits with positive gain, best gain: -inf
[LightGBM] [Warning] No further splits with positive gain, best gain: -inf
[LightGBM] [Warning] No further splits with positive gain, best gain: -inf
[60] valid_0's multi_logloss: 0.779169
[LightGBM] [Warning] No further splits with positive gain, best gain: -inf
[LightGBM] [Warning] No further splits with positive gain, best gain: -inf
[LightGBM] [Warning] No further splits with positive gain, best gain: -inf
[LightGBM] [Warning] No further splits with positive gain, best gain: -inf
[LightGBM] [Warning] No further splits with positive gain, best gain: -inf
[LightGBM] [Warning] No further splits with positive gain, best gain: -inf
[LightGBM] [Warning] No further splits with positive gain, best gain: -inf
[LightGBM] [Warning] No further splits with positive gain, best gain: -inf
[LightGBM] [Warning] No further splits with positive gain, best gain: -inf
[LightGBM] [Warning] No further splits with positive gain, best gain: -inf
[LightGBM] [Warning] No further splits with positive gain, best gain: -inf
[LightGBM] [Warning] No further splits with positive gain, best gain: -inf
[LightGBM] [Warning] No further splits with positive gain, best gain: -inf
[LightGBM] [Warning] No further splits with positive gain, best gain: -inf
[80] valid_0's multi_logloss: 0.771128
[LightGBM] [Warning] No further splits with positive gain, best gain: -inf
[LightGBM] [Warning] No further splits with positive gain, best gain: -inf
[LightGBM] [Warning] No further splits with positive gain, best gain: -inf
[LightGBM] [Warning] No further splits with positive gain, best gain: -inf
[LightGBM] [Warning] No further splits with positive gain, best gain: -inf
[LightGBM] [Warning] No further splits with positive gain, best gain: -inf
[LightGBM] [Warning] No further splits with positive gain, best gain: -inf
[LightGBM] [Warning] No further splits with positive gain, best gain: -inf
[LightGBM] [Warning] No further splits with positive gain, best gain: -inf
[LightGBM] [Warning] No further splits with positive gain, best gain: -inf
[LightGBM] [Warning] No further splits with positive gain, best gain: -inf
[LightGBM] [Warning] No further splits with positive gain, best gain: -inf
[LightGBM] [Warning] No further splits with positive gain, best gain: -inf
[100] valid_0's multi_logloss: 0.76556
[LightGBM] [Warning] No further splits with positive gain, best gain: -inf
[LightGBM] [Warning] No further splits with positive gain, best gain: -inf
[LightGBM] [Warning] No further splits with positive gain, best gain: -inf
[LightGBM] [Warning] No further splits with positive gain, best gain: -inf
[LightGBM] [Warning] No further splits with positive gain, best gain: -inf
[LightGBM] [Warning] No further splits with positive gain, best gain: -inf
[LightGBM] [Warning] No further splits with positive gain, best gain: -inf
[LightGBM] [Warning] No further splits with positive gain, best gain: -inf
[LightGBM] [Warning] No further splits with positive gain, best gain: -inf
[LightGBM] [Warning] No further splits with positive gain, best gain: -inf
[LightGBM] [Warning] No further splits with positive gain, best gain: -inf
[LightGBM] [Warning] No further splits with positive gain, best gain: -inf
[120] valid_0's multi_logloss: 0.760782
[LightGBM] [Warning] No further splits with positive gain, best gain: -inf
[LightGBM] [Warning] No further splits with positive gain, best gain: -inf
[LightGBM] [Warning] No further splits with positive gain, best gain: -inf
[LightGBM] [Warning] No further splits with positive gain, best gain: -inf
[LightGBM] [Warning] No further splits with positive gain, best gain: -inf
[LightGBM] [Warning] No further splits with positive gain, best gain: -inf
[LightGBM] [Warning] No further splits with positive gain, best gain: -inf
[LightGBM] [Warning] No further splits with positive gain, best gain: -inf
[LightGBM] [Warning] No further splits with positive gain, best gain: -inf
[LightGBM] [Warning] No further splits with positive gain, best gain: -inf
[LightGBM] [Warning] No further splits with positive gain, best gain: -inf
[LightGBM] [Warning] No further splits with positive gain, best gain: -inf
[LightGBM] [Warning] No further splits with positive gain, best gain: -inf
[LightGBM] [Warning] No further splits with positive gain, best gain: -inf
[LightGBM] [Warning] No further splits with positive gain, best gain: -inf
[LightGBM] [Warning] No further splits with positive gain, best gain: -inf
[LightGBM] [Warning] No further splits with positive gain, best gain: -inf
[LightGBM] [Warning] No further splits with positive gain, best gain: -inf
[LightGBM] [Warning] No further splits with positive gain, best gain: -inf
[140] valid_0's multi_logloss: 0.757663
[LightGBM] [Warning] No further splits with positive gain, best gain: -inf
[LightGBM] [Warning] No further splits with positive gain, best gain: -inf
[LightGBM] [Warning] No further splits with positive gain, best gain: -inf
[LightGBM] [Warning] No further splits with positive gain, best gain: -inf
[LightGBM] [Warning] No further splits with positive gain, best gain: -inf
[LightGBM] [Warning] No further splits with positive gain, best gain: -inf
[LightGBM] [Warning] No further splits with positive gain, best gain: -inf
[LightGBM] [Warning] No further splits with positive gain, best gain: -inf
[LightGBM] [Warning] No further splits with positive gain, best gain: -inf
[LightGBM] [Warning] No further splits with positive gain, best gain: -inf
[160] valid_0's multi_logloss: 0.754428
[LightGBM] [Warning] No further splits with positive gain, best gain: -inf
[LightGBM] [Warning] No further splits with positive gain, best gain: -inf
[LightGBM] [Warning] No further splits with positive gain, best gain: -inf
[LightGBM] [Warning] No further splits with positive gain, best gain: -inf
[LightGBM] [Warning] No further splits with positive gain, best gain: -inf
[LightGBM] [Warning] No further splits with positive gain, best gain: -inf
Early stopping, best iteration is:
[171] valid_0's multi_logloss: 0.752807
하이퍼파라미터가 튜닝된 LGBM 모델 정확도: 0.7018140589569161
'머신러닝 > 머신러닝: 심화 내용' 카테고리의 다른 글
머신러닝 앙상블 기법 2 : 스태킹(Stacking) (1) | 2024.12.27 |
---|---|
머신러닝 앙상블 기법 1 : 보팅(Voting) (0) | 2024.12.26 |
피처 선택(Feature Selection) (0) | 2024.12.24 |
피처 중요도(Feature Importance) (0) | 2024.12.24 |
피처 생성(Feature Generation) 2 : 다항식 피처 생성 (0) | 2024.12.24 |